Business intelligence and analytics have transformed how organizations approach problem-solving and future planning. These essential tools allow companies to sift through large volumes of data to uncover actionable insights, leading to smarter and more efficient decision-making. Let’s explore how businesses utilize these technologies to enhance their decision-making processes and drive growth.
The Role of Business Intelligence in Business Decision Making
Business intelligence (BI) refers to the technologies, tools, and processes used to collect, organize, analyze, and exhibit an organization’s raw data in a meaningful and actionable way.
Primarily, BI relies on analyzing historical and current data to provide insights that guide business operations and strategies. BI tools gather and clean data from various sources, analyze it, and display results through reports, dashboards, and visualizations tailored for decision-makers.
Incorporating BI into your strategy allows for informed decisions, trend identification, and improved efficiency. By shifting from reactive to proactive decision-making, BI ensures choices are rooted in data-driven insights.
The Role of Business Analytics in Strategic Business Planning
Business Analytics (BA) applies techniques like statistical analysis, data mining, predictive modeling, and machine learning to interpret data and extract actionable insights for strategic planning.
It focuses on anticipating future trends and making decisions to influence outcomes. BA enables businesses to evaluate the potential impact of their choices, forecast scenarios, and implement strategies more effectively. This predictive approach empowers organizations to make smarter, data-driven decisions and enhances the likelihood of successful outcomes.
Comparing Business Analytics with Business Intelligence
Business Analytics (BA) and Business Intelligence (BI) are complementary data management approaches that provide different insights:
- Business Intelligence examines past and current data to show what has happened and is happening.
- Business Analytics uses predictive models to anticipate future trends and outcomes.
BI provides a foundation for BA, enabling accurate forecasts and proactive strategies. Together, they give a comprehensive view of an organization, supporting informed decisions and strategic planning. Integrating both tools boosts agility and competitiveness in dynamic environments like market shifts and regulatory compliance.
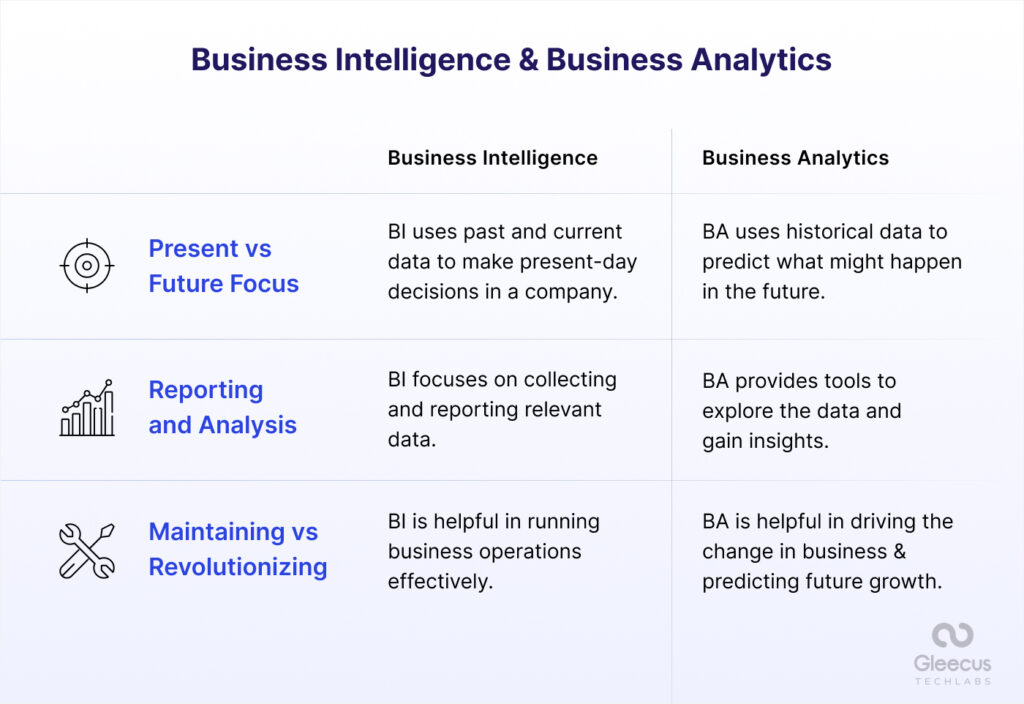
How Can You Transform Your Enterprise Data Into Actionable Insights?
You can convert raw, unstructured data into meaningful insights by following these four steps:
Data Collection
Identify and gather the right data that aligns with your business goals. This foundational step sets up efficient data source management.
Data Integration
Clean and process the data to meet quality standards. Utilize DataOps practices to ensure reliability and accessibility.
Data Analysis
Use Business Analytics to uncover trends, solve problems, and identify opportunities for growth and improved customer service.
Data Visualization
Present insights visually using tools like Tableau or Power BI, enabling informed, strategic decision-making.
Business Intelligence (BI) simplifies complex company data into comprehensible reports, metrics, and trends, enabling managers to make informed decisions.
Real-time Business Intelligence (RTBI) takes this further by analyzing live data and presenting actionable visual insights in the moment.
How Predictive Analytics (Predictive Power of BA) Benefits Business Processes Across Industries?
The predictive power of business analytics significantly influences decision-making across various sectors. Check out this detailed look at how predictive analytics impacts decision-making in various industries:
Retail
Demand Forecasting: Predictive analytics enables retailers to accurately forecast customer demand by analyzing historical sales data, market trends, and consumer behavior. Walmart optimizes its inventory levels based on past sales data to ensure there is no stockouts or overstocking situations.
Personalized Marketing: Personalization in retail marketing involves segmenting customers based on purchasing behavior and preferences, and tailoring marketing campaigns to enhance engagement. Predictive analytics involves analyzing customer data and designing targeted promotions for specific customer groups.
Pricing Optimization: Retailers can use predictive analytics to assess the impact of sales frequency on customer behavior, allowing them to set prices that maximize sales while maintaining profitability.
Finance
Risk Management: Banks employ predictive models to assess credit risk and likelihood of repayment for loan underwriting. Borrower’s historical financial data and current financial expense pattern is analyzed to discover this.
Fraud Detection: Predictive analytics helps detect fraudulent activities by identifying unusual patterns in transaction data that may indicate fraud. Credit card companies use predictive algorithms to monitor transactions in real-time and flag suspicious activities for further investigation.
Investment Strategies: Investment firms leverage predictive analytics to analyze historical stock performance and forecast market trends and optimize investment portfolios based on projected returns and risks.
Manufacturing
Supply Chain Optimization: Predictive analytics allows manufacturers to forecast demand for products accurately, enabling better planning of production schedules and inventory management.
Maintenance Scheduling: Predictive maintenance models analyze equipment performance data to predict failures before they occur, reducing downtime and maintenance costs. Siemens implements predictive maintenance strategies that utilize sensor data from machinery to schedule maintenance proactively.
Quality Control: Manufacturers use predictive analytics to identify factors that contribute to defects in production processes, allowing for timely interventions. Toyota employs predictive analytics in its quality control processes to monitor production lines for anomalies that could lead to defects.
Energy & Utilities
Demand Forecasting: Utilities use predictive analytics to forecast energy demand based on historical consumption patterns and external factors such as weather conditions. Pacific Gas and Electric uses predictive models to anticipate peak usage times, enabling better resource allocation during high-demand periods.
Grid Management: Predictive analytics helps utilities optimize grid operations by predicting outages or equipment failures before they happen. Duke Energy employs predictive analytics tools to monitor grid health and predict when maintenance is needed.
Renewable Energy Integration: Utilities leverage predictive models to forecast solar and wind energy demand based on weather forecasts, optimizing energy distribution accordingly. This plays an important role in grid management.
Healthcare
Patient Outcome Predictions: Hospitals employ predictive models to identify patients at high risk for readmission, allowing for targeted interventions post-discharge.
Resource Allocation: Predictive analytics helps healthcare facilities optimize staffing levels and resource allocation based on anticipated patient volumes. Cleveland Clinic uses predictive models to forecast patient admissions, enabling better scheduling of staff and resources.
Clinical Decision Support: AI-driven predictive tools assist clinicians in making informed decisions about patient care by analyzing clinical data in real-time. Merative utilizes predictive analytics to provide evidence-based treatment recommendations based on patient data.
Life Sciences
Drug Development Optimization: Predictive analytics accelerates drug discovery by identifying potential candidates through analysis of biological data and chemical properties. Insilico Medicine uses generative AI combined with predictive analytics for rapid identification of promising drug compounds.
Patient Stratification in Clinical Trials: Predictive models help identify suitable candidates for clinical trials based on genetic and demographic factors. Companies like Foundation Medicine leverage predictive analytics to match patients with clinical trials that align with their genetic profiles.
Market Access Strategy Development: Pharmaceutical firms analyze market trends using predictive models to forecast the success of new drug launches in specific demographics. Life sciences companies use predictive insights to develop effective market access strategies for new therapies.
How BI Promotes Real-time Decision Making
BI plays a crucial role in enhancing organizational performance by providing tools and insights that facilitate informed decision-making. Here’s how BI benefits businesses across various aspects of a business.
Business Metrics Report
Data-Driven Insights: BI tools aggregate and analyze data from various sources to generate comprehensive business metrics reports. These reports provide key performance indicators (KPIs) that help organizations monitor their performance against strategic goals.
Easy Sharing of Business Insights
Collaboration and Transparency: BI platforms enable easy sharing of insights across departments, fostering collaboration and ensuring that all stakeholders have access to relevant information. A marketing team can share campaign performance dashboards with sales and finance departments, ensuring everyone is aligned on goals and results, which enhances cross-departmental collaboration.
Rapid Decision Making
Real-Time Data Access: BI tools provide real-time access to data analytics, allowing decision-makers to respond quickly to changing market conditions or operational challenges. A manufacturing company can use BI dashboards to monitor production metrics in real-time, enabling managers to make immediate adjustments to optimize efficiency and reduce downtime.
Identifying Sales Opportunities
Predictive Analytics: BI systems often incorporate predictive analytics capabilities that help identify potential sales opportunities by analyzing customer behavior and market trends.
Quick Review
Dashboards and Visualizations: BI tools offer intuitive dashboards that present complex data in easily digestible formats, allowing for quick reviews of performance metrics. Executives can review key financial indicators through a visual dashboard at a glance during board meetings, facilitating faster discussions and decision-making.
Operational Efficiency
Process Optimization: By analyzing operational data, BI helps organizations identify inefficiencies in processes and recommend improvements.
Conclusion
AI and machine learning are transforming business intelligence (BI) by enhancing forecasts and data analysis. Augmented analytics democratizes BI, enabling users at all levels to grasp complex data. The growing adoption of real-time data empowers businesses and customers to respond swiftly to changes.
As data volume surges, robust data governance and security become critical. These advancements profoundly influence business strategies and operational efficiency.
Gleecus TechLabs Inc. leads this transformation by delivering tailored BI and data analytics solutions.
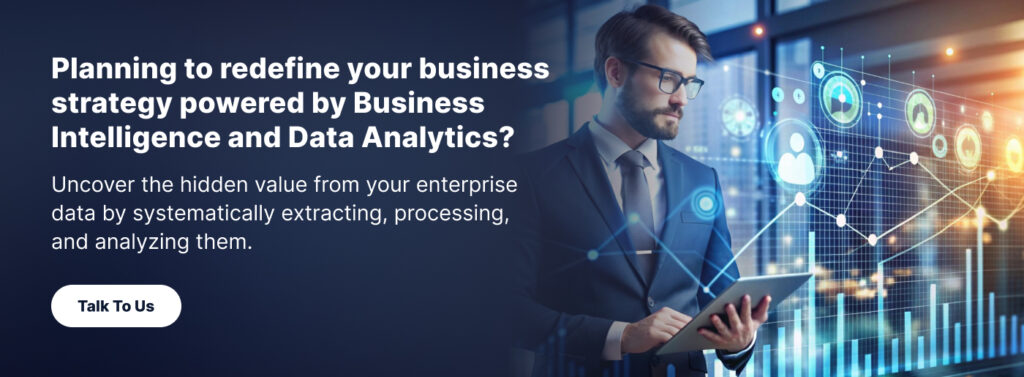
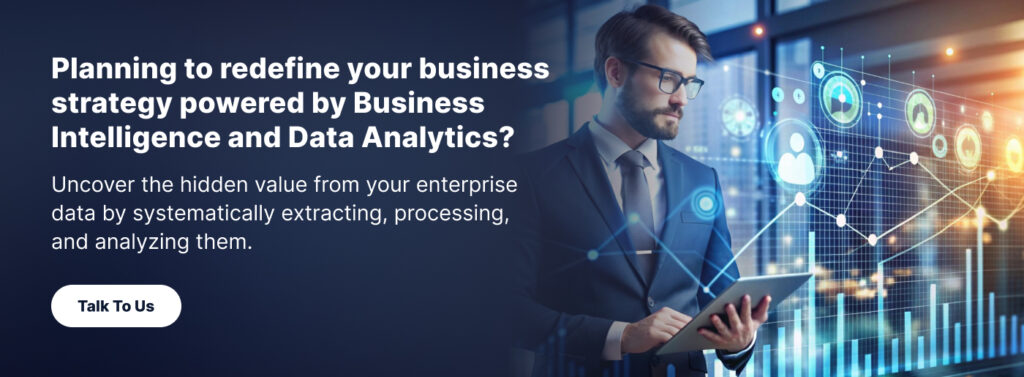