With their vast resources, wealth of proprietary data, and often fluid business models, banks have naturally led the charge in adopting machine and deep learning technologies. Over the years, AI in banking has proven invaluable in enhancing risk management, preventing losses, fighting fraud, and strengthening customer loyalty. Today, AI has become a core driver of efficiency and profitability, making it a cornerstone of modern banking operations.
The application of machine learning in banking accelerated in the late 2000s with the development of Python for Data Analysis, or pandas–an open-source data analysis package written for the Python programming language. Pandas, along with other machine learning software libraries, like SKLearn and TensorFlow, made data structuring and analysis easier, more systematic, and thus opened the door to more accessible machine learning algorithms and powerful analytical frameworks.
Bank | AI Application |
---|---|
Barclays | The bank has an AI-tool that seeks to prevent fraud by predicting potential instances using real-time monitoring of merchant payment transactions. |
Banco Santander | An AI-tool, called Kairos shows how a corporate client could be impacted by economic events, creating prediction patterns that enable employees to make more informed investment and lending decisions. |
Bank of America | A platform, called Glass, helps sales and trading employees uncover hidden market patterns to anticipate client needs by consolidating market data across asset classes and regions with the bank’s inhouse models and machine learning techniques. |
JP Morgan | The bank has applied to trademark a tool called IndexGPT that could act as a financial investment advisor. |
How AI in Banking Improves Creditworthiness
The banking sector is a regulated services industry that relies heavily on technology. By actively integrating AI for efficiency, service enhancement, and risk management, banks can strengthen their credit quality, highlighting AI’s powerful role in reshaping the financial sector’s resilience and stability.
Well deployed AI could enhance operating revenues, by improving employees’ decision-making and by unlocking the revenue potential of clients–not least due to personalized services and products. And there could be a significant positive effect on costs, resulting from a robust AI strategy that reduces operating expenses, and thus improves efficiency and profitability.
Shifts in these factors can be relevant in assessment of a banks’ business position, notably where they exacerbate the difference between banks’ competitive position by contributing to stronger franchises, and more agile and profitable business models.
Generative AI in banking promises to exacerbate these differences by also playing a role in banks’ ability to upscale and modernize legacy IT systems–notably with low-code /no-code software that could offer important savings. This could also reduce operational risks and costs that arise from running banks on old infrastructure and labor-intensive systems. It is estimated that a 10% reduction in bank staff costs would, on average, improve return-on-equity by about 100 basis points and cost-to-income ratios by about 3 percentage points, based on S&P Global Ratings’ Global Top 200 rated banks. These potential gains can be balanced against the investment in AI tech that they require.
AI also has the potential to enhance risk management and could thus influence the view of a bank’s risk profile, albeit indirectly. For example, in credit risk, a bank that can accurately price risk and use patterns hidden in data to determine the likelihood customers will repay debt (or become problematic) will improve its workout models, reduce problematic loans, and improve the accuracy of its provisioning. In effect, its risk-adjusted performance should improve, relative to peers’.
There are numerous ways that AI could be used to enhance risk management practices in banking or improve other operational areas. Let’s dive into them.
Use Cases of AI in Banking
Risk Management
Banks are prone to risk from external factors like currency fluctuations, natural disasters, and political unrest. Having a clear, formalized risk management plan brings additional visibility into consideration. AI/ML powered risk management solutions offer improved forecasting accuracy and perform richer data segmentation for analysis.
Customer Experience
As Generative AI is gaining popularity for enterprise banking operations it tends to transform how banks communicate with customers. With AI-driven virtual assistants, banks can anticipate customer needs before they are even expressed. These assistants can provide real-time, personalized solutions, helping customers navigate complex banking processes, such as loan applications or investment decisions, with ease. Capital One has an AI assistant, Eno, who can handle various customer inquiries, from checking account balances to reviewing transaction histories. Generative AI-powered Agent Assist aids agents in adjusting response through sentiment analysis and enhances agents’ knowledge base by analyzing vast data.
Loan Approval
The Global AI in lending market is expected to be worth around USD 58 billion by 2033, growing at a CAGR of 23.5%. Traditional loan approval goes through a number of document processing. Document processing software leverages a new technology, IA (Intelligent Automation), a blend of AI and automation. ML algorithms, trained on historical data, adeptly recognize document structures, extract relevant information, and categorize content. An AI-based system rates a new customer’s creditworthiness by looking into relevant metrics such as current income level, monthly average spending, and employment opportunities going beyond traditional scoring system like FICO and increasing the pool of eligible loan customers.
Financial and Market Predictions
Tracking market involves processing large volumes of data and AI with its underlying, powerful data analysis algorithms uncover unprecedented insights. AI systems can identify complex correlations and construct sophisticated predictive models. AI algorithms analyze a myriad of factors, including macroeconomic indicators, geopolitical events, and consumer sentiment, to forecast market trends and guide investment decisions. This also helps the product team to anticipate changing preferences and spending patterns and come up with new financial product offers.
Cybersecurity and Fraud Detection
Innovation in banking technologies and normalization of digital experiences have generated new attack vector for fraudsters and cyber criminals. From identity theft, Business Email Compromise (BEC) to Authorized Push Payment (APP) Fraud via Phishing digital banks are regularly being challenged with some fraud attempt. AI-powered document processing software verifies KYC for a loan applicant and scans through submitted account information to detect anomalies and reduce false positives. NLP and ML analyze data such as past transactions, fraud accidents, and risk parameters the lender sets and awards a fraud score. Strong cybersecurity measures are crucial for the sake of goodwill and compliance. Gen AI can track transactions, analyze patterns, and flag anomalies that suggest fraudulent activity. By continuously updating its detection algorithms, Gen AI can stay ahead of evolving fraud schemes, ensuring that banks maintain strong defenses. Gen AI use cases in banking also extend to biometric verification and multi-factor authentication (MFA) powered by AI chatbots.
AI can be used to ensure compliance with anti-money laundering (AML) regulations.ML models can analyze alternative data sources and, by understanding natural language, flag critical
cases automatically.
Micro-Savings and Product Recommendation
Everyday spending habits often hide opportunities for small yet impactful savings. Generative AI automates micro-savings strategies, to generate custom packages, savings plans, and debt repayment schedules tailor to your financial needs. Generative AI tailors investment strategies based on your unique risk tolerance and long-term financial vision. It goes a step further by considering how you emotionally respond to market fluctuations, ensuring your investments truly align with your personal financial journey, not just market trends.
How Banks Should Approach AI
The IBM Institute for Business Value published a guide for banks looking to embed AI tools and practices into their operations. Some of the key actions are:
Establishing a clear AI governance and risk profile of the bank
Bank leaders must make informed decisions on AI deployment, recognizing that robust security measures are essential to counter any associated risks. Embracing AI with this proactive approach ensures that banks leverage its benefits while safeguarding against potential vulnerabilities.
Prioritize use cases
Focus on specific use cases when deploying AI, ensuring they align with organizational goals and deliver tangible results. Examples of impactful applications include customer-facing chatbots, personalized investment solutions, fraud detection, and credit scoring models.
Choose a trusted AI platform
Enterprise AI solutions often rely on integrating multiple models to meet organizational needs. Banks must decide between using open-source models, in-house-built models, or a combination of both to ensure they are equipped for success.
Learn from initial deployments
Banks concerned about risks should implement small-scale tests and use cases to assess the impacts before scaling and deploying new implementations. Early lessons are valuable because they help banks better understand what other infrastructure they need to deploy and where they need to make adjustments.
Establish an AI Factory
Once an organization has established a workable strategy for building or adopting AI for specific use cases, it should build an apparatus that adds AI to its operations and makes it central to all development and business methods.
Conclusion
AI is transforming banking with hyper-personalized customer recommendations to lender profile review. As more financial institutions adopt AI-powered tools, the banking sector will continue to evolve, offering customers unparalleled convenience, security, and personalization. The integration of AI in banking has become imperative for institutions seeking to thrive in an increasingly competitive and dynamic market
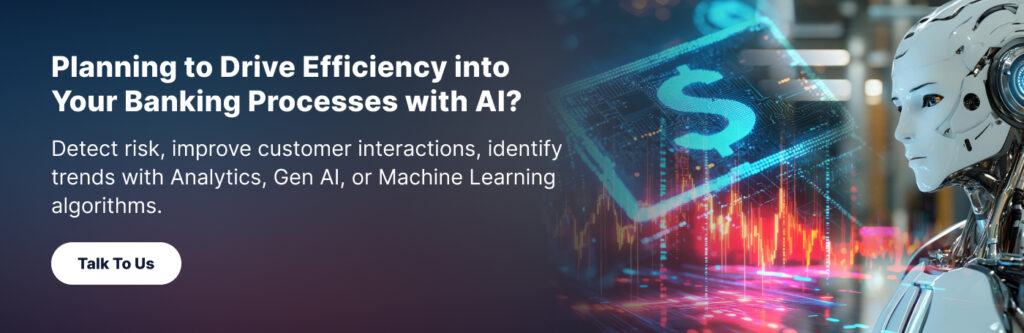