The rise of grid-connected devices like EV charging stations and residential solar panels in the energy sector increases the unpredictability of energy flow across the grid. Cloud-connected grid gives rise to the number of datapoints available for analyzing electricity demands and tracking decarbonization efforts. According to a study by Greenbyte AB, annually the global fleet of wind turbines produces more than 400 billion data points per year. AI serves as an important solution for tackling and analyzing this surge in data by the grid and its connected devices.
AI (Artificial Intelligence) as a technology can process tons of grid generated data within a short period of time. Predictive capabilities of AI enables energy businesses to analyze current data, compare with historical data, and predict demand in power or condition of electrical devices. The self-learning capability of ML (machine learning), a subset of AI ensures that it trains on historical data and helps with grid optimization or energy trading. There are several interesting applications of AI in the energy sector the following section discusses some of them.
Applications of AI in the Energy Sector
Smart grids
AI can help manage smart grids, by predicting consumption patterns using historical and real-time data. Which in term helps to optimize resource allocation. AI enables to anticipate sudden surge in electricity demand beforehand and plan distribution to prevent blackouts. In case of a grid disruption AI algorithms can automatically reroute power to minimize service interruptions, reduce downtime, and improve grid reliability.
Demand response management
Demand Response Management (DRM) is a core strategy of the energy sector for grid stability. This strategy involves initiating loadshedding to adjust electricity usage of consumers when signaled by grid operators and energy providers. The strategy aims at maintaining sanctity of the grid during peak periods. By utilizing AI, DRM can optimize and control electricity consumption in response to demand fluctuations.
DRM involves incentivizing shift or shed in electricity demand in wholesale or ancillary power markets. A key example is of ABB, a Swiss manufacturer that allows commercial building managers to avoid peak charges and benefit from time-of-use tariffs with its AI-enabled energy demand forecasting application.
Predictive maintenance
ML trains on historical data, maintenance logs, etc. to predict potential breakdown of an equipment. Utility company E.ON has developed a machine learning algorithm to predict when medium voltage cables in the grid need to replaced. ML armed with RPA (robotic process automation) can even propose a maintenance schedule as a preemptive measurer to minimizes downtime, reduces repair costs, and improves the overall reliability of energy infrastructure. A predictive asset analytics solution helped Tata Power, a 17000+ MW energy generation infra owner, move from reactive to proactive maintenance by analyzing asset data for accurate equipment condition assessment. This helped the company to gain advanced warning of emerging equipment issues, avoid asset failures, and reduce unscheduled maintenance.
Renewable energy forecasting
Energy companies and governments are quite enthusiastic about renewable energy due to their sustainable and eco-friendly nature. The catch is, renewable energy sources like hydel power, wind, or solar are unpredictable governed by the laws of nature. AI algorithms analyze weather forecasts, historical generation data, and real-time conditions to predict the availability of renewable energy sources or impending disruption.
Google and its AI subsidiary DeepMind developed a neural network in 2019 to increase the accuracy of forecasts for its 700 MW renewable fleet. Based on historical data, the network developed a model to predict future output up to 36 hours in advance with much greater accuracy than was previously possible.
Do you plan to ramp up your renewable energy production capacity with highly accurate predictive ability? Consult us on how AI solutions can be your gamechanger?
Energy storage
AI optimizes the storage and distribution of energy from renewable sources. Battery energy storage systems (BESSs) are a great way to secure a consistent flow of energy in case of sudden shortages in the source. With algorithms monitoring and determining the likes of demand, supply, price and grid conditions, AI can guide businesses as to the best times to store energy, when to release it, and how much to distribute. Siemens have introduced a virtual power plant (VPP) leveraging digital twins technology. It is a representation of the full power infrastructure virtually, including the physical assets and control systems. This has turned out to be a significant energy storage system supporting the next level of energy optimization for Finnish brewery Sinebrychoff.
Carbon Capture, utilization, and storage (CCUS)
AI enhances the efficiency of CCUS processes by optimising the capture of carbon dioxide from the atmosphere or emission sources. AI-driven systems can identify the most suitable methods for utilising captured carbon, whether for industrial processes or safe long-term storage. This technology plays a vital role in reducing greenhouse gas emissions and mitigating climate change.
A case study report by Geoteric explores the feasibility of using AI networks to rapidly and accurately screen potential CCUS sites, specifically focusing on the Southern North Sea Triassic Bunter Sandstone. The detailed AI fault analysis indicates the selected site has minimal faults, making it a promising location for CCUS purposes.
Energy trading
AI with its predictive abilities offers excellent solutions for energy trading. It offers lightning-fast decisions to execute numerous trades in milliseconds. Its pattern recognition ability serves as an excellent lead to detect market opportunities and risks that may elude human traders. AI optimizes energy portfolios, simulates market scenarios, analyses sentiment, automates tasks, and continually adapts to changing market conditions. It processes real-time data on pricing, demand, and supply trends, enabling energy companies to make informed and profitable trading decisions.
Smart homes and buildings
The impact of AI on homes and buildings is nothing short of transformative in the pursuit of energy efficiency as AI transforms them into energy-efficient ecosystems. Smart meters and IoT devices work in harmony with AI to create intelligent, responsive ecosystems. These systems continuously monitor energy consumption in real-time, allowing AI to make data-driven decisions that optimize energy utilization.
Oil and gas exploration
AI is revolutionizing the oil and gas exploration sector by analyzing vast geological data, identifying hidden reserves, and improving exploration success rates. It enhances efficiency, reduces resource waste, and lowers costs. AI’s applications extend to reservoir analysis, drilling optimization, anomaly detection, and safety monitoring, while also helping to reduce emissions and promote sustainability across the industry. This technological integration transforms exploration, production, and the environmental footprint of the oil and gas sector.
Baker Hughes, a global leader in industrial turbomachinery and innovation, developed the Leucipa automated field production solution, harnessing AWS’s advanced analytics and Baker Hughes’s domain expertise. This solution empowers operators to autonomously and proactively manage field production and energy efficiency, maximizing value from producing wells, minimizing operational downtime, and optimizing overall well performance.
Nuclear power plant monitoring
AI’s role in nuclear power plants is indispensable, as it ensures the highest levels of safety and helps prevent accidents AI systems are designed to maintain a vigilant watch over every aspect of plant operations, operating 24/7 without fatigue. These systems continuously analyze data from various sensors and instruments, detecting even the slightest anomalies or deviations from established safety standards. Through advanced predictive maintenance models, AI goes beyond identifying issues; it anticipates potential equipment failures by assessing data such as performance trends, wear and tear, and operational stresses.
Conclusion
To thrive in the changing energy environment and maximize business value companies need to adopt AI-integrated solutions like predictive maintenance, digital twins, energy forecasting. At Gleecus, we help enterprises accelerate their decision-making and streamline workflows powered by AI. Our team trains and upskills your workers to get familiarity with new AI tools and practices to maximize the values of your AI initiatives.
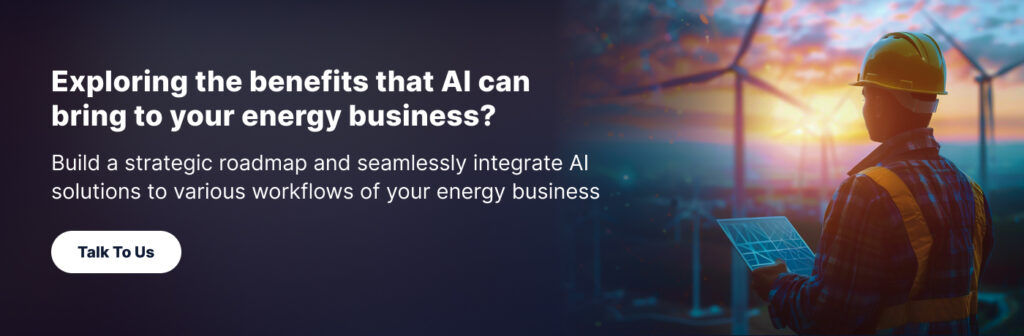