The healthcare sector is navigating one of its most significant evolutions, powered by Artificial Intelligence (AI). As global healthcare systems confront aging populations, rising costs, clinician shortages, and an ever-expanding volume of medical data, AI emerges as a powerful ally. It is not merely enhancing efficiency—it is reimagining the way care is delivered, diseases are diagnosed, and treatments are developed.
AI in healthcare involves a confluence of technologies such as machine learning, computer vision, and natural language processing. These systems can analyze complex datasets, generate predictive insights, and support medical professionals in delivering faster, more accurate, and personalized care. This blog explores the key components, use cases, benefits, challenges, and future trends surrounding AI in healthcare.
The Core Technologies Powering AI in Healthcare
1. Machine Learning (ML)
Machine learning lies at the heart of AI in healthcare. ML models are trained on large volumes of clinical data—ranging from electronic health records (EHRs) to genetic profiles—to uncover patterns and make data-driven predictions. These models are applied in areas such as:
- Predictive analytics for patient outcomes
- Risk stratification for chronic diseases
- Anomaly detection in diagnostics and lab results
Supervised, unsupervised, and reinforcement learning techniques each play unique roles, from classifying medical conditions to learning optimal treatment paths.
2. Natural Language Processing (NLP)
A substantial portion of clinical data exists in unstructured formats like physician notes, discharge summaries, and pathology reports. NLP enables machines to interpret, extract, and structure this information. Use cases include:
- Clinical documentation automation
- Summarization of patient histories
- Extraction of key symptoms and medication patterns
NLP helps streamline workflows, improve documentation accuracy, and enhance decision-making by converting free-text data into actionable insights.
3. Computer Vision
Medical imaging is a critical domain where AI excels. Computer vision algorithms can analyze X-rays, MRIs, CT scans, and histopathological slides with high precision. These systems can detect minute anomalies that may be missed by the human eye and support early intervention in conditions like tumors, fractures, and organ abnormalities.
Key capabilities include:
- Automated image segmentation
- Pattern recognition for disease classification
- Quantification of changes over time for monitoring
4. AI-Powered Robotics
Robotic systems enhanced with AI are increasingly being integrated into surgical, rehabilitative, and caregiving roles. In surgery, these systems can perform minimally invasive procedures with precision, while in physical therapy, robotic devices adapt to patient progress using feedback loops.
Features include:
- Real-time motion tracking
- Predictive force modulation
- Adaptive assistance in rehabilitation protocols
5. Generative AI
Generative models are used to create synthetic medical data, simulate patient outcomes, and assist in drug design. These systems enable:
- Generation of diverse clinical trial scenarios
- Design of novel molecular structures for therapeutics
- Simulation of disease progression in virtual environments
Such capabilities drastically shorten research timelines and reduce costs in pharmaceutical development and clinical planning.
Major Use Cases of AI in Healthcare
1. Enhanced Diagnostics
AI models can rapidly analyze diverse data points—medical images, biomarkers, patient histories—and identify disease patterns with high accuracy. Unlike traditional diagnostic tools that rely on fixed rule sets, AI systems learn continuously from new data inputs, improving their performance over time.
Outcomes include:
- Earlier detection of complex diseases
- Reduced diagnostic errors
- Support for differential diagnosis
2. Personalized Medicine
AI enables hyper-personalized care by integrating genetic, behavioral, and environmental data. This approach shifts treatment strategies from a one-size-fits-all model to highly individualized therapies. By understanding a patient’s unique biology and lifestyle, AI can:
- Tailor medication dosages
- Predict treatment response
- Minimize side effects and drug interactions
3. Drug Discovery and Development
AI accelerates pharmaceutical research by predicting how potential compounds interact with biological targets. Deep learning models simulate protein folding, molecular binding, and pharmacokinetics to identify the most promising candidates.
Key benefits:
- Reduced R&D timelines
- Improved success rates in clinical trials
- Cost savings in early-phase screening
4. Remote Monitoring and Virtual Health
With the proliferation of wearables and home health devices, AI can continuously track patient vitals and flag abnormalities in real time. Machine learning models analyze signals such as heart rate, oxygen levels, and sleep patterns to detect signs of deterioration or recovery.
Advantages:
- Early intervention before emergencies
- Improved chronic disease management
- Reduced need for hospital visits
5. Workflow and Operational Optimization
Beyond clinical care, AI automates repetitive administrative tasks that traditionally consume a significant portion of healthcare professionals’ time. Natural language processing and robotic process automation (RPA) can:
- Automate billing and claims processing
- Streamline appointment scheduling
- Extract structured data from clinical notes
This not only reduces operational costs but also enhances the patient and clinician experience.
Key Benefits of AI Integration in Healthcare
1. Improved Accuracy and Efficiency
AI systems analyze vast amounts of data far more quickly than human practitioners and uncover subtle patterns that may elude traditional methods. This results in:
- Faster turnaround times for diagnostics
- Lower error rates
- More precise treatment plans
2. Cost Reduction
AI reduces expenditures across the care continuum by minimizing unnecessary testing, optimizing resource allocation, and reducing manual labor. It enables hospitals and clinics to operate more efficiently under constrained budgets.
3. Expanded Access to Care
AI-driven virtual care and diagnostic tools can be deployed in remote or underserved areas. This democratizes access to high-quality medical services without requiring patients to travel long distances.
4. Enhanced Research Capabilities
By automating data extraction and modeling, AI dramatically accelerates biomedical research. It enables:
- Faster hypothesis testing
- Real-time cohort identification
- Insight discovery from heterogeneous data sources
Challenges and Considerations
1. Data Privacy and Security
AI systems must process sensitive health information, making robust cybersecurity essential. Healthcare organizations must implement encryption, access controls, and anonymization techniques to comply with regulations like HIPAA and GDPR.
2. Algorithmic Bias
If AI models are trained on incomplete or unrepresentative datasets, they may exhibit biases that lead to disparities in diagnosis or treatment. Addressing bias requires:
- Diverse and inclusive training data
- Regular auditing of model outputs
- Transparent model design and validation
3. Regulatory and Legal Barriers
AI tools that influence clinical decisions are often subject to regulatory scrutiny. Developers must navigate complex approval pathways and establish clear guidelines for liability and accountability in AI-assisted care.
4. Interoperability and Integration
Many healthcare facilities use legacy systems with limited compatibility. Integrating AI requires robust APIs, data standardization, and cross-platform compatibility to ensure seamless operation without disrupting clinical workflows.
Future Trends in AI-Driven Healthcare
As artificial intelligence becomes an essential pillar in modern healthcare, the future will be defined by intelligent, collaborative, and increasingly autonomous systems. These evolving technologies are not just improving clinical efficiency—they are fundamentally redefining how care is accessed, delivered, and experienced.
Explainable AI (XAI)
With AI systems making clinical predictions and treatment recommendations, trust is critical. Explainable AI (XAI) models offer transparency into how these decisions are made. Instead of opaque “black box” outputs, XAI allows healthcare providers to understand, audit, and validate algorithmic behavior. This transparency is key to ensuring accountability in high-stakes environments like diagnostics, triage, and medication management.
Edge AI in Healthcare
Edge AI processes medical data at the source—on wearable devices, bedside monitors, or mobile diagnostic tools—reducing latency and improving responsiveness in critical care. For example, edge-based AI in ambulances or rural clinics can enable real-time stroke or cardiac assessments even before a patient reaches the hospital, helping frontline responders make timely interventions.
Federated Learning for Collaborative AI
To protect patient privacy while maximizing the value of data, federated learning enables machine learning models to be trained across multiple healthcare institutions without sharing raw data. This is particularly useful for training diagnostic models across rare disease datasets, pediatric populations, or geographically diverse patient groups—ensuring equity, accuracy, and confidentiality.
AI Agents: The Future Backbone of Healthcare Systems
AI agents represent one of the most transformative trends in healthcare technology. Unlike traditional models that perform isolated tasks, AI agents are goal-driven systems that can reason, plan, act autonomously, and collaborate across domains. In the context of healthcare, they are poised to become the intelligent intermediaries between patients, providers, and digital systems.
Conclusion
AI is not just a technological tool—it is a transformative force in the healthcare domain. From diagnostics to treatment, from research to operations, AI is enhancing efficiency, accuracy, and accessibility across the board. While the journey to full-scale integration involves addressing significant challenges, the benefits are too compelling to ignore.
As AI matures and becomes more explainable, equitable, and integrated, it will redefine the future of healthcare—delivering smarter, faster, and more humane care for all.
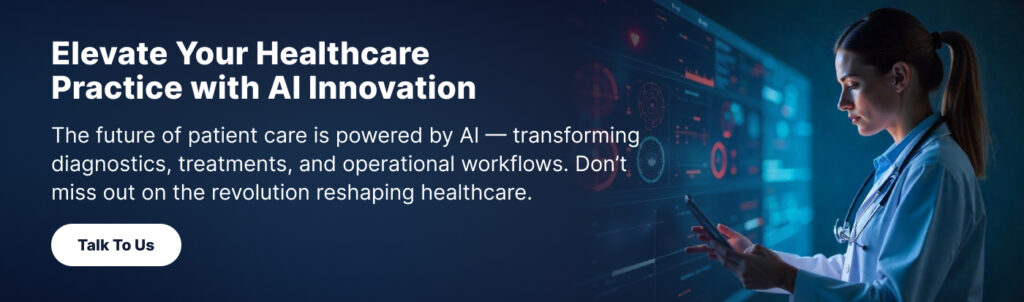