The development of AI/ML models involves a complex series of steps, including data collection, preprocessing, model training, and evaluation. Automation plays a pivotal role in accelerating AI/ML development by minimizing manual intervention and streamlining processes.
Artificial Intelligence (AI) and Machine Learning (ML) are at the forefront of technological innovation, enabling systems to learn from data and make intelligent decisions. The development of AI/ML models involves a complex series of steps, including data collection, preprocessing, model training, and evaluation. As organizations increasingly rely on data-driven insights, the demand for efficient and effective model development has surged.
Automation plays a pivotal role in accelerating AI/ML development by minimizing manual intervention and streamlining processes. Automated Model Training not only enhances productivity but also democratizes access to machine learning capabilities, allowing organizations of all sizes to harness the power of AI. According to MarketsandMarkets, the AutoML market is projected to reach USD 6.4 billion by 2025, reflecting its growing significance in the tech landscape.
Understanding Automated Machine Learning
What is Automated Machine Learning (AutoML)?
Automated Machine Learning (AutoML) refers to the use of software tools that automate the end-to-end process of applying machine learning to real-world problems. This technology enables users—regardless of their technical expertise—to build high-performing models efficiently. For instance, PayPal utilized H2O.ai’s AutoML system to enhance fraud detection capabilities, increasing model accuracy from 0.89 to 0.947—a remarkable 6% improvement that accelerated their development timeline by six times.
Key Components of Automated Model Training
Automated model training encompasses several critical components:
Data Preparation
Data preparation is foundational for effective model training. Automated tools streamline data extraction, cleaning, and transformation processes, ensuring high-quality input for models.
Feature Engineering
Feature engineering involves creating new features from raw data to improve model performance. For example, California Design Den experienced a 50% reduction in inventory carryover after utilizing Google Cloud AutoML for e-commerce predictive analytics. This automation allowed them to respond quickly to market fluctuations.
Model Selection
Selecting the right algorithm is essential for achieving optimal results. AutoML platforms automate this process by evaluating multiple algorithms and configurations to identify the best-performing model based on predefined criteria.
Hyperparameter Optimization
Hyperparameter optimization is a critical aspect of model training that involves fine-tuning parameters to enhance performance. Automation in this area allows for systematic exploration of various combinations to find the most effective settings for each model.
Validation and Testing
Validation ensures that models are not overfitting by using separate datasets during training. Automated systems perform validation as part of the training process, allowing for continuous assessment and adjustment of model performance.
Evaluation Metrics
To assess model performance accurately, various evaluation metrics—such as accuracy, precision, and recall—are utilized. Automated tools provide insights into these metrics, helping users understand how well their models are performing.
Who can benefit from using AutoML?
Automated Machine Learning (AutoML) provides significant advantages across various sectors, enabling a broad range of users to benefit from its capabilities. Here are the key groups that can gain from using AutoML:
Data Scientists and AI Engineers
Efficiency Enhancement: AutoML automates repetitive tasks such as data preprocessing, model selection, and hyperparameter tuning, allowing data scientists to focus on more complex problems and innovative solutions.
Improved Productivity: By reducing the time spent on manual tasks, data scientists can produce high-performing models more quickly, leading to faster project completion and deployment.
Businesses of All Sizes
Small and Medium Enterprises (SMEs): AutoML democratizes access to machine learning by enabling non-experts to create models without extensive technical knowledge. This is particularly beneficial for SMEs that may lack dedicated data science teams.
Large Corporations: Larger organizations can leverage AutoML to streamline their operations, enhance decision-making processes, and maintain a competitive edge in their respective markets.
Non-Technical Users
Business Analysts and Marketers: With user-friendly interfaces, AutoML tools allow professionals without a deep understanding of machine learning to implement predictive analytics in their workflows. This accessibility fosters innovation across departments.
Decision Makers: Executives can utilize AutoML for forecasting and trend analysis, enabling them to make informed decisions based on data-driven insights without needing to understand the underlying algorithms.
Industries with Data-Driven Needs
Healthcare: AutoML can analyze medical records and predict patient outcomes, helping healthcare professionals improve treatment strategies and operational efficiency.
Finance: Financial institutions use AutoML for fraud detection, risk assessment, and customer segmentation, enhancing their ability to respond to market changes rapidly.
Retail: Retailers benefit from AutoML in inventory management, customer behavior prediction, and personalized marketing strategies, allowing for optimized operations and improved customer engagement.
Manufacturing: AutoML aids in predictive maintenance and supply chain optimization, helping manufacturers reduce costs and improve productivity through better resource management.
Educational Institutions
Researchers and Students: AutoML tools can be used in academic settings to facilitate machine learning education. They allow students to experiment with models without needing extensive programming skills, making learning more accessible.
How AutoML Works
Pipeline Automation
AutoML systems create automated workflows that encompass all stages of model development—from data preparation through deployment—ensuring a seamless transition between each phase.
Algorithm Selection and Evaluation
AutoML platforms iterate through various algorithms and configurations in parallel, evaluating each model’s performance against specified metrics until the optimal solution is identified.
Real-Time Feedback and Adjustments
Automated systems provide real-time feedback during model training, allowing for immediate adjustments based on performance metrics. This iterative approach enhances overall model quality.
Tools and Technologies for Automated Model Training
Popular AutoML Platforms
Azure Machine Learning: Offers a comprehensive suite for building, training, and deploying machine learning models with robust AutoML capabilities.
Google Cloud AutoML: Provides tools tailored for different types of machine learning tasks, enabling users to create custom models with minimal coding experience.
H2O.ai: Known for its open-source platform that supports a variety of algorithms for automated machine learning tasks.
Best Practices for Implementing Automated Model Training
Defining Clear Objectives
Establishing clear goals before starting an AutoML project ensures alignment with business needs and helps measure success effectively.
Selecting the Right Data Sets
Choosing high-quality datasets is crucial for training effective models. Organizations should ensure that their data is representative of real-world scenarios.
Monitoring and Evaluating Models Post-Deployment
Continuous monitoring post-deployment is essential to maintain model performance over time. Organizations should regularly evaluate their models against new data to adapt to changing conditions.
Challenges and Considerations
Limitations of Automation in AI/ML
While automation significantly enhances efficiency, it may not always produce the best results compared to expert-tuned models. Users must balance automation with human oversight to ensure optimal outcomes.
Balancing Automation with Human Insight
Human expertise remains vital in interpreting results and making strategic decisions based on model outputs. A collaborative approach between automated systems and human analysts can yield the best results.
Future Trends in Automated Model Training
Emerging Technologies in AI/ML Development
As technology evolves, new advancements in AI/ML will continue to enhance automated processes. Innovations such as quantum computing may further accelerate model training capabilities.
The Role of AI in Enhancing Automation
Artificial intelligence itself will play a pivotal role in refining automation processes within machine learning development, leading to even more efficient workflows.
Conclusion
Automated Model Training represents a significant leap forward in AI/ML development by streamlining complex processes while enhancing accuracy and efficiency. As organizations increasingly embrace automation technologies, they position themselves to harness the full potential of AI-driven solutions.
The success stories from companies like PayPal, Walmart, Pfizer, and Airbus illustrate how AutoML can transform operations across various industries. Embracing automation in AI/ML development not only accelerates project timelines but also democratizes access to advanced analytics capabilities across industries. Organizations should consider integrating AutoML into their workflows to stay competitive in an evolving digital landscape.
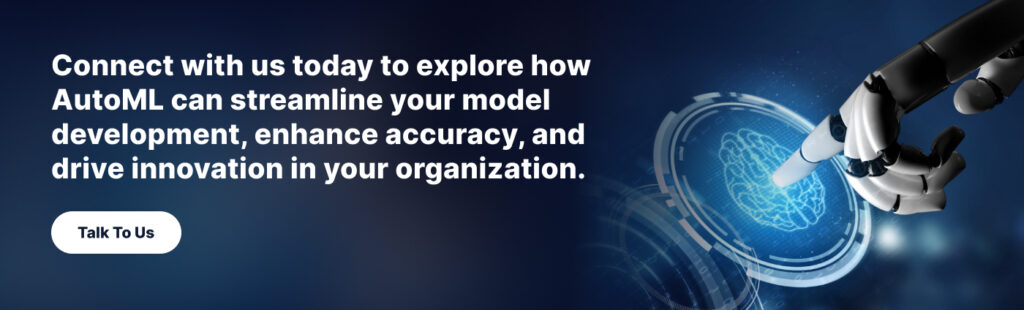