The global business intelligence market is predicted to grow at 9% CAGR to USD 63.76 billion by 2032. BI is turning from a nice-to-have tools to a driver of strategy, operations, and growth for organizations. Data is the new oil for enterprises and BI tools enables to analyze the raw data and uncover valuable insights. This article explores the top trends that are going to rule the innovation and implementation of BI engines in 2025.
1.Augmented Analytics
Augmented Analytics is an advanced approach to data analytics that leverages AI and ML to enhance data preparation, insight generation, and sharing of insights across organizations.
Data preparation and processing
To unlock the full potential of their BI platforms, organizations need to analyze, clean, standardize, and integrate vast amounts of raw data. AI streamlines these ETL (extract, transform, and load) processes, ensuring BI teams work with accurate, relevant, and properly formatted data while significantly reducing time and effort.
Moreover, AI takes it a step further by uncovering valuable insights hidden within unstructured data that were previously inaccessible.
Personalization
AI-powered BI tools deliver personalized insights tailored to each user’s preferences and roles. By analyzing behavior and past data interactions, AI customizes reports, highlights key metrics, and provides context-rich insights, making the experience more intuitive and contextual.
Natural language interaction
Business intelligence empowers more employees across an organization by making data accessible. NLP (Natural Language Processing) pushes this further by enabling no-code users to explore data independently. A ML technology, NLP allows computers to understand, process, and generate human language seamlessly.
NLP creates a user-friendly experience in a BI platform. It lets individuals analyze data simply by asking questions in human language. This “Generative BI” approach interprets queries, performs analysis, and delivers answers in human-friendly language. By eliminating the dependency on BI engineers and data scientists, NLP ensures a smooth and effortless path to uncovering insights.
2. Self-service Business Intelligence
Relying on IT teams or business analysts often creates bottlenecks in the business intelligence process, delaying insights for decision-makers.
Self-service BI tools eliminate these delays by empowering users across all functions to act as citizen data scientists. They can access, analyze, and act on business data independently, reducing dependence on IT or BI specialists.
These tools feature intuitive interfaces that make navigating data simple. Users can explore visualizations with drag-and-drop features, create customized reports, and set up alerts with ease. By promoting data literacy and democratizing analytics, self-service BI enhances data discovery and ensures stakeholders can uncover valuable insights effortlessly.
3. Embedded Analytics
Embedding BI components like visualizations and reports directly into everyday workflows and applications is a growing trend in business intelligence. This approach integrates insights seamlessly into existing tools rather than requiring a separate platform.
Embedded analytics supports:
- Ease of Adoption: Embedded analytics deliver BI within familiar apps and environments, aligning with existing workflows instead of creating new ones.
- Boosted Productivity: Generating insights directly from known tools reduces the learning curve, enhances performance, and speeds up value realization.
- Enhanced Collaboration: Integrated analytics bring BI to all stakeholders, fostering seamless insight sharing and data-driven discussions within core applications.
This approach ensures BI becomes a natural part of daily operations, driving smarter, faster decisions.
4. Data Governance
BI tools process vast amounts of business data to deliver actionable insights. As self-service BI expands and operates across multiple cloud environments, the risks of unauthorized access, misuse, and distrust in data grow. Ensuring data security, quality, and integrity is essential for optimizing BI outcomes and complying with regulations like CCPA and GDPR.
Effective data governance involves defining clear ownership and usage policies, using encryption, and enforcing role-based access controls. It also includes overseeing how AI interacts with BI data, ensuring transparency in predictive analytics, and maintaining up-to-date business contexts for AI forecasts.
As businesses increasingly rely on AI-driven analytics, robust data governance will be critical to unlocking value while safeguarding trust and compliance.
5. Data Quality Management
A BI solution depends entirely on data quality to deliver accurate insights for decision-making. As a result, data quality management has become a critical element of BI deployments, with the market for these tools expected to grow to $8.49 billion by 2030.
Poor data quality in BI can lead to wasted investments, flawed analytics, bad decisions, and compromised strategies. It also weakens generative AI applications by reducing their effectiveness and decision intelligence.
Data quality management tools cleanse, standardize, verify, and align datasets while validating data sources. They provide the solid foundation needed for reliable, data-driven business strategies.
6. Collaborative BI
Collaborative BI integrates teamwork tools into business intelligence systems to improve data sharing, communication, and decision-making across teams.
Platforms like Power BI combined with Microsoft Teams make it easy to share insights and foster collaboration across departments. These tools encourage participation from various stakeholders, allow teams to analyze data together, use integrated discussion threads, and solve problems collectively, promoting data-driven decisions and fostering a data-driven culture within the organization. Key Features of Collaborative BI include:
Shared dashboards and reports
Collaborative BI platforms enable users to create and share dashboards and reports that provide a unified view of data. This allows teams to access the same information, fostering alignment and informed discussions. Team members can clearly see contributions and insights from others, promoting accountability for decisions made based on collective analysis.
Real-Time collaboration
Users can collaborate in real-time on data analysis, making it easier to discuss findings, interpret results, and make decisions based on the most current information.
Commenting and annotations
Many collaborative BI tools allow users to add comments or annotations directly on visualizations or reports. This feature facilitates discussions around specific data points or trends, enhancing the context of the analysis.
Version control
Collaborative BI systems often include version control features that track changes made to reports or dashboards. This ensures that all team members are working with the most up-to-date information and can revert to previous versions if needed.
Integration with communication tools
Collaborative BI solutions frequently integrate with communication platforms (e.g., Slack, Microsoft Teams) to streamline discussions about data insights, making it easier for teams to share findings and insights.
Access control and permissions
Collaborative BI platforms typically offer granular access control settings, allowing organizations to define who can view or edit specific reports or dashboards. This ensures data security while promoting collaboration.
7. Decision Intelligence
Decision Intelligence (DI) is an emerging branch of BI that enhances decision-making by combining data analytics, AI, and ML into a unified framework. DI improves decision quality and speed by delivering actionable, data-driven insights.
Using ML algorithms, DI processes big data, applies a semantic layer of business rules, and generates predictive insights to support smarter, forward-looking decisions.
8. Mobile BI
Mobile BI is becoming increasingly important for business leaders as it empowers them with real-time access to critical data and insights while on the go. With the widespread use of smartphones and the rise of BYOD (bring your own device) policies, mobile-optimized BI solutions are becoming more common.
Cloud BI growth and faster mobile internet speeds have made interactive, mobile-friendly business intelligence a practical and appealing reality.
9. Data Storytelling
Most major BI platforms now feature data storytelling extensions. Data storytelling adds narrative structure to BI visualizations, highlighting key insights and explaining what’s happening and why.
It creates a clear data narrative that connects visualizations, guiding audiences instead of leaving them to infer insights. This is especially helpful when sharing data with diverse teams who might otherwise overlook important trends.
10. Process Intelligence
A recent HFS research study found that 88% of enterprise leaders expect to increase investments in Process Intelligence. Business Intelligence and Process Intelligence are closely related, complementary, and increasingly intersecting practices. Process Intelligence helps BI professionals turn insights into action by identifying the “how,” “why,” and “where” of process optimization to meet business goals. By adding process insights to BI, Process Intelligence enables leaders to spot value opportunities, target processes for improvement, and act swiftly and effectively.
Conclusion
These trends highlight the dynamic nature of Business Intelligence as it adapts to technological advancements and evolving business requirements. By embracing these changes, organizations can enhance their analytical capabilities, foster a culture of data-driven decision-making, and ultimately gain a competitive advantage in their respective markets.
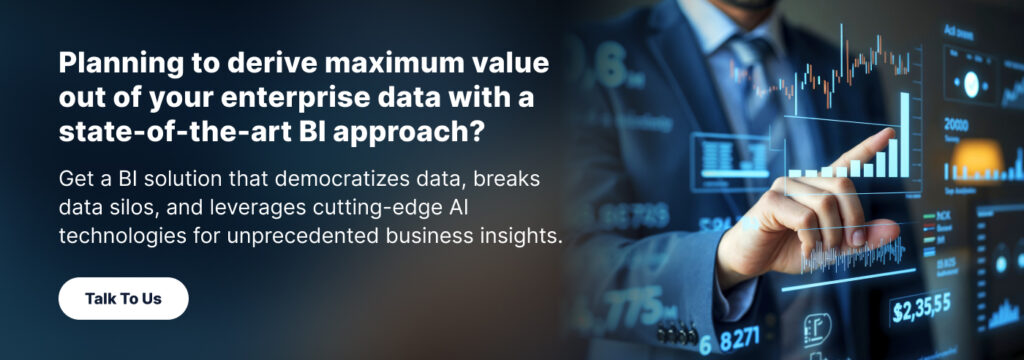