Retailers traditionally relied on instincts and experience to make critical decisions about inventory, pricing, and location. However, in today’s competitive market with narrow profit margins and rapidly changing consumer preferences, intuition alone is insufficient. Data analytics enhances decision-making in retail businesses by providing precise, data-driven insights. This technology helps retailers optimize their strategies, ensuring they can meet demand accurately, manage inventory efficiently, and adjust prices effectively. By integrating data analytics in retail, businesses can refine their instincts and make more profitable decisions in a complex market environment.
Benefits of Data Analytics in Retail
Data analytics in retail leverages a set of tools that help retailers to reduce cost, improve margins, and increase revenue. Following is some of the ways data-driven retail analytics helps businesses:
Inventory management
In the face of cutthroat competition in retail, failure to close a customer because of inventory stock out is detrimental to customer satisfaction and revenue. At the same time overstocking a product that is losing demand challenges the retailer to clear inventory with steep discounts and taking a loss. This problem is very evident in sectors like fashion where demand rapidly changes. Data Analytics in retail supports predictive analytics to forecast what product is trending in and what is going out of demand. Retailers can stock up their inventory and revise sales strategy based on that.
Personalized Recommendation
Customer experience is the glue to customer loyalty and personalization helps with that. Data analytics in retail takes the game of personalization to the next level with personalized recommendation. Analyzing a customer’s social media interactions and product browsing history helps ML models to analyze customer sentiment and recommend products with increased chance of closure.
Improving pricing decisions
Data about various factors like abandoned shopping carts, competitive pricing information, and the cost of goods helps retailers to figure out the optimal price. Prices are dynamically changed following trends to maximize profits and minimize losses. Pricing decisions can also be individualized depending on what a particular customer adds to his cart and offer special pricing for related products to increase the chance of upselling and cross selling at the same time delight customers.
Systematic order management
Retail doesn’t end with just being able to sale a product in fact, in the era of COD the product is not sold until delivered. Analytics helps to determine bottlenecks in order preparation and delivery path. Metric like order preparation time and carrier performance helps to plan for fast order delivery. Data analytics driven BI suites helps businesses to track order cancellations, preparation times and delivery promises. With a holistic view of order management, stock points, items and delivery promises, businesses can adapt to changing market dynamics with agility.
Types of Data Analytics in Retail
Retail data analytics can be categorized into four main types: descriptive, diagnostic, predictive, and prescriptive. Each type serves a unique purpose in understanding and optimizing business operations.
Descriptive analytics
This involves gathering and analyzing historical data to identify trends and patterns. It answers questions like “what happened?” but doesn’t delve into the reasons. For instance, it might show that customer satisfaction scores have dropped but not explain why.
Diagnostic analytics
This type goes deeper to uncover the reasons behind trends identified by descriptive analytics. By examining data sources like customer feedback and social media, diagnostic analytics can reveal that poor satisfaction scores are due to long wait times in customer service.
Predictive analytics
Using historical data, predictive analytics forecasts future behaviors and trends. For example, it can predict when a customer might need to reorder a product, helping businesses proactively address customer needs and reduce churn.
Prescriptive analytics
This advanced form of analytics not only predicts future outcomes but also recommends actions to achieve desired results. For instance, it might suggest that reducing resolution times by 20% could boost customer retention by 50%. It combines AI and big data to provide actionable insights for decision-making.
These analytics types collectively help retailers enhance their operations, improve customer satisfaction, and drive business growth by transforming data into strategic insights.
Application of Data Analytics in Retail
Companies use data analytics in retail to explain past operational and financial performance, diagnose what might have gone wrong, suggest alternative approaches for store associates, customer service agents, and others involved with retail business.
In-store analytics
In-store analytics involves collection of data from POS systems and in-store video cameras to track customer behavior and interactions within physical retail spaces. Data analytics on in-store data helps to gauze customer experience based on metrics like foot traffic, dwell time, and product interactions. This in turn helps VMs (Visual Merchandizers) to plan the inventory distribution for an engaging experience. Camera data also helps to identify the bottlenecks in inventory stocking or movement and any areas exposed to shop-lifting. POS data helps to understand customer preferences, and areas from where maximum goods get sold this in turn helps to evaluate the success of VM strategy.
Customer analytics
Tracking customer preference involves collecting data from systems that customers interact with, including POS systems, websites, phone logs, and customer service chats. This data helps to build omnichannel experiences for customers. Customer analytics in turn helps to create personalized experiences, improve customer engagement, boost customer retention, and increase company revenue.
Inventory analytics
Inventory analytics share insights about your gauge the movement and performance of your goods. This involves tracking inventory related metrics like inventory carrying cost, inventory turnover ratio, backorder rate, stockout rate etc. It helps to spot outliers, patterns, or certain correlations in your inventory data. Based on inventory analytics retailers can maintain healthy stock levels. This also helps to reduce inventory holding cost and track the reasons of inventory shrinkage weighing to theft, shipping damages, and wrong inventory accounting.
Web analytics
Web analytics is key to the measuring the success of e-commerce businesses. Collecting data for web analytics involves tracking metrics like cart abandonment rate, average order value, and the average number of products per sale. Based on web analytics demand forecasting, sales forecasting, and supply chain planning happens. It also helps to track unusual activities on the web and prevent fraud. For example, with a policy in place to return money a fraudulent customer may complain about damage or missing products very frequently or almost every time he orders. Web analytics can identify such customers and blacklist them.
Business intelligence
Data analytics in retail is at the heart of BI (Business Intelligence) tools and dashboards. BI involves leveraging data analytics to identify trends and patterns. This in turn helps with better decision making with regards to inventory, pricing, marketing, and sales. BI tools like Power BI are used for data mining, text analytics, dashboards and visualizations. Business intelligence helps in understanding customer behavior, improving merchandizing, developing effective campaigns, and planning inventory management.
Data Sources for Data Analytics in Retail
Data analytics for retail gather data from various sources. The following are some of the common sources:
Point-of-sale systems (POS)
These systems track and manage customer transactions, providing data on purchases. They also generate sales and customer trend reports which serve as data for powerful BI tools.
Customer relationship management (CRM) software
Applications in this category manage sales, marketing, customer service, and e-commerce processes. Retailers use CRM to track customer interactions, retain individual customer data, and identify potential sales and marketing opportunities.
Inventory management systems
This software tracks stock levels, monitors inventory in warehouses and distribution centers, and forecasts demand. It helps identify optimal storage locations to minimize transportation costs and meet customer demand efficiently.
Retailers use this software to track items in stock, monitor inventory levels in warehouses and distribution centers, and create forecasts of demand. It also helps retailers identify optimal locations for storing certain items to minimize transportation expenses and ensure that goods are available to meet customer demand.
Conclusion
In the near future, data analytics in retail will seamlessly integrate into everyday operations, becoming both ubiquitous and inconspicuous. Much like smartphones’ constant use of location data, analytics will be continuously leveraged by users and applications, often without their awareness. For business professionals, analytics will shift from generating weekly reports to being an intrinsic part of their daily workflow. Access to AI-driven insights will be widespread, subtly enhancing regular business activities. This normalization will render AI in retail leveraging data analyses a standard, rather than a novelty.
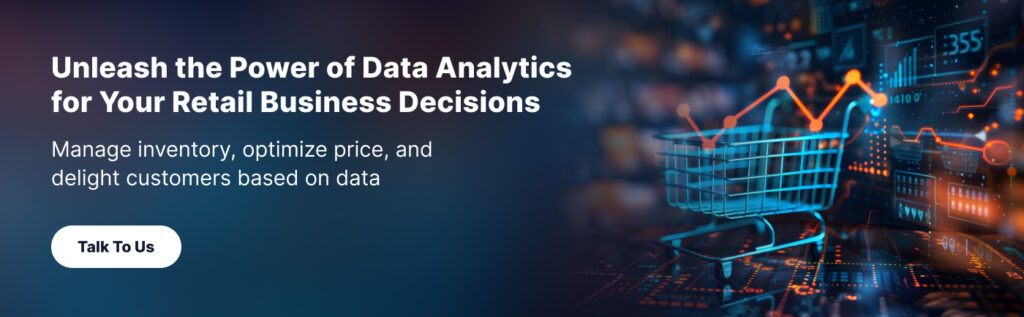