If your business is investing in AI automation, here’s a hard truth: AI is only as good as the data it runs on. And if you don’t have visibility into that data — where it’s coming from, how it’s changing, and whether you can trust it — you’re flying blind.
You may already have monitoring tools in place. But traditional monitoring is reactive — it catches issues after they’ve already impacted performance.
That’s where data observability comes in. Data observability is proactive. It offers a high-level view and deep diagnostic insight into how your data is behaving across systems, models, and automation workflows – in real-time. Observability surfaces anomalies that can quietly derail your AI efforts.
Think of it as operational clarity for your AI initiatives. When you can see what your data is doing, you can trust what your AI is doing. And in today’s fast-moving business environment, that level of visibility isn’t optional — it’s strategic.
The 5 Pillars of Data Observability for Reliable AI
For AI automation to deliver on its promises, your data must be trustworthy. The five core pillars of data observability form the foundation of a transparent, resilient, and scalable AI system.
1. Freshness: Is Your Data On Time?
In AI, fresh data is critical. Outdated or delayed data can lead to decisions based on irrelevant information. Observability tools track data freshness in real time.
2. Volume: Is Your Data Spiking or Dropping?
AI systems rely on the consistency of data volume to make accurate predictions. Observability tools provide visibility into data volume patterns, detecting unexpected spike or drop in data volume.
3. Schema: Are You Working with the Right Data Structure?
Changes in data schema — like new fields or modified data types — can break models or lead to inaccurate insights. Data schema observability helps you track these changes in real time.
4. Lineage: Where Is Your Data Coming From?
Without data lineage, you’re left guessing where data has been, how it’s been transformed, and whether it’s reliable. Data observability helps you track the path of your data from source to destination.
5. Distribution: Is Your Data Consistent Across Time?
The consistency of data distributions is key to maintaining stable AI models. Observability into data distribution tracks how data changes over time.
Why Data Observability Matters for AI Automation
Here’s why data observability is a game-changer for your AI automation strategy:
AI Automation Is Only as Good as the Data Behind It
If the data feeding your AI is outdated, inconsistent, or erroneous, even the best models will fail.
By integrating data observability into your workflow, you can:
- Track data freshness to make sure models are working with the most current information.
- Monitor data consistency across sources and pipelines, preventing errors from propagating through your AI systems.
- Identify anomalies in real time that could negatively impact decision-making or model performance.
The Risk of Automation Without Observability
Without data observability, your AI automation is a black box. You may not know why your models are underperforming or whether your data is even reliable. This lack of transparency introduces significant risks:
- Unreliable outputs: If your models are fed poor-quality data, your automation will make flawed decisions — potentially harming business outcomes.
- Model degradation: Over time, AI models can degrade if they aren’t retrained on fresh, accurate data. Data observability helps you catch these issues early, allowing you to update models before they cause real damage.
- Pipeline failures: Data pipeline errors can go unnoticed without proper monitoring, leading to disruptions that affect your entire automation system.
Why Transparent Data Is Essential for Your Business
As AI plays a larger role in your decision-making, ensuring data transparency becomes crucial. Data observability offers you a detailed view of how data is flowing through your systems, helping you:
- Build trust in your AI systems, knowing that they are powered by high-quality, accurate data.
- Comply with regulations in data-sensitive industries by maintaining full traceability of your data pipeline.
- Enhance decision-making with the confidence that your automation is based on dependable and transparent data.
Proactive Management vs. Reactive Fixes
In the world of AI automation, waiting for issues to arise is risky and costly. Instead of scrambling to fix problems when they already affect your business, you can:
- Detect data issues early and take action before they impact model performance.
- Prevent errors from flowing into your automation, ensuring smoother operations and more reliable outputs.
- Iterate faster by continuously monitoring and improving the health of your data, enabling your AI systems to evolve with minimal disruption.
Now that we’ve established why data observability is foundational to AI automation, let’s explore how it directly impacts the performance of our machine learning models.
How Data Observability Improves Machine Learning Performance
In the world of AI automation, the quality of your data directly impacts the performance of your machine learning models.
Ensuring Consistency for Accurate Predictions
For machine learning models to perform at their best, they need consistent, high-quality data.
With data observability in place, you can:
- Monitor data consistency to prevent drift in model predictions.
- Ensure that AI is trained on real-time, relevant data, which leads to quicker, more accurate decision-making.
- Identify any issues in your data pipeline before they have a chance to impact model performance.
Accelerating Model Retraining with Proactive Insights
AI models need constant training and adjustments to stay relevant in dynamic environments. Data observability takes the guesswork out of tradtional model retraining approaches. It proactively helps you spot emerging issues in data quality or trends.
By tracking the health of your data, you can:
- Detect changes in data patterns that could indicate when a model needs retraining.
- Automate alerts for shifts in data distributions, ensuring you stay ahead of potential model degradation.
- Reduce downtime and increase the speed of model iteration, so your AI systems remain competitive and efficient.
Improving Model Explainability and Trust
A critical aspect of AI deployment, especially in regulated industries, is model explainability. With data observability, you gain complete visibility into the data pipeline, making it easier to trace the origins of any decision a model makes.
Observability ensures that:
- You have visibility into every data point that feeds into your AI models, improving interpretability.
- Your models can be audited easily, and you can explain the reasoning behind every automated decision.
- You can quickly trace and correct issues in the data that affect model outputs, ensuring transparency and accountability.
Industry-Specific Applications of Data Observability for AI Automation
Let’s explore how organizations across various industries can harness data observability to enhance their AI automation efforts.
1. Healthcare: Enhancing Predictive Models with Real-Time Data Monitoring
In the healthcare sector, timely and accurate data is paramount. Data observability can be integrated into a AI-driven patient monitoring system to enable:
- Real-time data monitoring, ensuring patient data like lab results and medical histories were current.
- Immediate anomaly detection, identifying discrepancies in patient records promptly.
- Improved predictive accuracy, leading to better patient care outcomes and reduced errors.
2. Finance: Streamlining Fraud Detection with Transparent Data Pipelines
Financial institutions rely heavily on AI for fraud detection. Banks leverage data observability tools to for:
- Continuous monitoring of transaction data to spot unusual patterns indicative of fraud.
- Alert systems that flagged data inconsistencies before they affected model predictions.
- Enhanced model performance, reducing false positives and improving customer trust.
3. E-commerce: Personalizing Customer Experience with Data Transparency
E-commerce platforms thrive on personalized customer experiences. Data observability allows refinement of recommendation engines leading to:
- Quick identification of data pipeline issues, minimizing disruptions in service.
- Consistent data quality, ensuring customer preferences and behaviors were accurately captured.
- Increased conversion rates, as product recommendations became more relevant and timely.
4. Manufacturing: Optimizing Predictive Maintenance with Accurate Sensor Data
In manufacturing, equipment downtime can be costly. Adopting Data observability leads to:
- Real-time monitoring of sensor data to detect anomalies early.
- Reduced maintenance costs, by addressing issues before they escalated.
- Improved operational efficiency, keeping production lines running smoothly.
5. Technology: Enhancing Data Reliability in AI Systems
Tech companies often deal with vast amounts of data. For example, Contentsquare and GitLab leveraged data observability to:
- Reduce the time data engineers spent on manual data quality checks.
- Implement automated monitoring, freeing up resources for more strategic tasks.
- Improve overall data reliability, enhancing the performance of their AI systems.
Conclusion: From Data Blind Spots to AI Brilliance
In an age where AI automation is no longer optional but essential, data observability is the foundation of effective, scalable, and reliable AI systems. By ensuring that the data feeding your AI is high quality, fresh, and consistent, you’re safeguarding your automation initiatives and setting up your business for success. It’s not just about improving AI performance — it’s about reducing risk, improving trust, and gaining a competitive edge in a data-driven world.
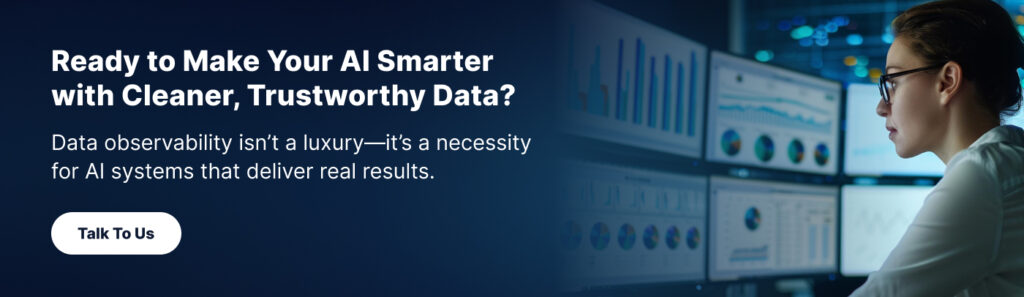