Businesses today compete to stay ahead of the curve with innovative solutions and cutting-edge technology. They optimize their processes and make data-driven decisions. This is where Enterprise AI Services come into play, offering a transformative approach to business operations. Enterprise AI provides entrepreneurs insights into business operations and market trends, helps in making future-oriented adjustments with predictive analytics, and plays a significant role in enterprise security.
The Rise of Enterprise AI Services
Enterprise AI is a blanket term used for referring to AI technologies that addresses any kind of business challenges within an organization. This comprises of a number of sub technologies like machine learning, deep learning, natural language processing (NLP), Generative AI, IA (Intelligent Automation), and other AI techniques that offer a number of business solutions.
The Power of Enterprise AI in Delivering Business Solutions
Here are some key benefits of leveraging AI in enterprise solutions:
Data-Driven Decision Making
Businesses today heavily rely on data to make their operational and business decisions. AI algorithms can accelerate data analysis of the sea of data businesses generate and handle today. It churns valuable insights that improves accuracy of forecasting and helps businesses to improve marketing approach, streamline resource allocation, and identify bottlenecks in different processes.
Improved Customer Experience
A very popular adaptation of AI, especially Gen AI is in innovating customer service experience. Gen AI enabled chatbots provide a humanlike response to customers delivering a personalized touch and improving satisfaction. ML a sub technology of AI also trains on customer related data collected from various data points to analyze customer sentiment and pop product recommendations which are more likely to get purchased.
Process Automation
RPA (Robotic Process Automation) automates processes that are defined by set rules. Combining AI into these automated workflows sets decision making capabilities into them. They can be used to replace mundane tasks like employee onboarding processes, or compliance checking based on rules.
Innovation and New Product Development
AI can analyze competitor strategies, consumer feedback, and market trends to find new business opportunities and improve product development procedures. As a result, businesses are able to respond to changing market demands and innovate more.
Predictive Analytics
Predictive analytics, powered by AI, enables businesses to forecast future trends, customer behavior, and market shifts. It also serves a crucial technology in manufacturing and supply chain industry helping with machine maintenance schedule to supply chain route planning.
Supply Chain Optimization
AI can optimize supply chain operations by predicting demand, reducing lead times, and minimizing inventory costs. This leads to a more efficient and cost-effective supply chain.
Cybersecurity
AI exhibits three crucial features that makes it a go-to technology for cybersecurity; ability to quickly analyze large amounts of data, identify patterns to detect anomalies, and automate repetitive processes. This allows AI to flag suspicious behavior and fraudulent activity in real-time, helping businesses protect themselves and their customers from financial losses and security breaches.
Challenges to AI Adoption
While AI can be a popular technology for digitally smart enterprises there are some obstacles to its quick adoption. We will look into some of them in the following section:
An Explosion of Data
The velocity, volume, and variety of data generated by today’s enterprises are immense. But as organizations accumulate increasingly large datasets, a problem arises: How do they store, evaluate, understand, communicate, and merge data into the insights that can help them make decisions? Because good quality data is crucial fodder for AI solutions. Modernizing infrastructure to support data quality management is cornerstone to AI initiatives of an enterprise.
Diverse Stakeholders
AI integration brings in change into organizational tech stack and practices. There is an initial cost associated with upgrading existing IT infrastructure, hiring skilled professionals, and discarding technologies and hardware which no longer fit into the new system of things. Enterprise AI projects also mean improving communication and collaboration between three teams who are the main stakeholders AI practitioners (developers, data scientists, AI engineers), enterprise IT (IT administrators, MLOps, DevOps), and lines of business (marketing and sales, customer support, operations). An effective change management guideline needs to be devised to keep these three teams aligned as the AI pipeline grows. The board members and investors also need to be convinced of the ROI from Enterprise AI proposals.
Integrations and Security
AI integrations will involve leveraging state-of-the-art SaaS solutions and even migrating application and data to third-party platforms. AI is a resource intensive technology requiring high compute power, lightening fast data transfer, and seamless scaling. This often obliges companies to take their enterprise data and applications to a public cloud provider like AWS or Microsoft Azure. Not many enterprises are comfortable with the idea of losing their control over data to a third-party provider. There is a fear about the adequate security measures provided by them. Sometimes even such transfer might even be barred by regulatory bodies especially witnessed in the case of fintech applications with stringent privacy norms.
Implementing AI at Enterprise Scale
AI adoption is on the rise across every industry and has outpaced predictions for growth. To get ahead of this, enterprises need to anticipate their future AI needs and transition current workloads. Whether you’re implementing your first AI project or integrating AI workloads into your infrastructure, here’s a look at how to set your AI journey up for success.
Identify One AI Use Case to Start with
Instead of initiating a large transition, start with one use case that can directly impact a business problem and garner executive support without budget challenges. When identifying a use case, keep some factors in mind: Can this problem be easily tackled with available data that can be mined for insights using AI algorithms? Will this use case scale to a larger problem your organization is trying to solve? Is the use case simple enough to perform and explain to your business partners?
Pilot an AI Project
Getting started with an AI project can involve many steps, from building a team to choosing the right tools, AI workloads, and infrastructure. Successful AI projects require forming a team of subject matter experts to support this new initiative, in addition to recruiting data scientists
and AI engineers. Make sure to choose AI tools that are performance-optimized and verified for your workloads to avoid inaccurate outcomes. Lastly, provide a robust, accelerated computing platform with an end-to-end software solution stack.
Simplify Proof of Concepts
When conducting a proof of concept that can be used to create a preproduction application, it’s important to optimize resources and
infrastructure. The most successfully scalable AI solutions will be those that can be folded into existing enterprise infrastructure. Organizations
can get started with minimal initial investment and leverage state-of-the-art AI software suites to simplify the development workflow.
Scale Incrementally
Successfully piloting an AI project is just the beginning. Scaling AI is the most elusive challenge for enterprises and will remain a work in progress as the complexity and scope of your projects grow. By gradually expanding your AI infrastructure and improving interoperability, you can unlock greater flexibility, optimize budgets, and maximize the return on your AI investment.
Continuously Improve AI Models and Processes
Innovating business processes with AI is a continuous journey of adoption, evaluation, and improvement. Set up automated systems for continuous deployment and testing of new AI features and processes. By continuously monitoring deployments to see the gaps in workflows and optimizing your AI processes, you’ll be poised to unlock AI’s vast potential in your organization.
Conclusion
AI implementation needs to address all the use cases that the organization may need. Whether your organization is adopting AI as a new workload or looking to build a future-proof AI environment, making confident design and purchase decisions can be daunting
Generative AI, a subset of artificial intelligence, is also becoming increasingly valuable for enterprises. This technology goes beyond traditional AI by creating new content, such as text, images, and even code. Businesses have been leveraging it to automate content creation, develop innovative marketing strategies, and enhance customer engagement.
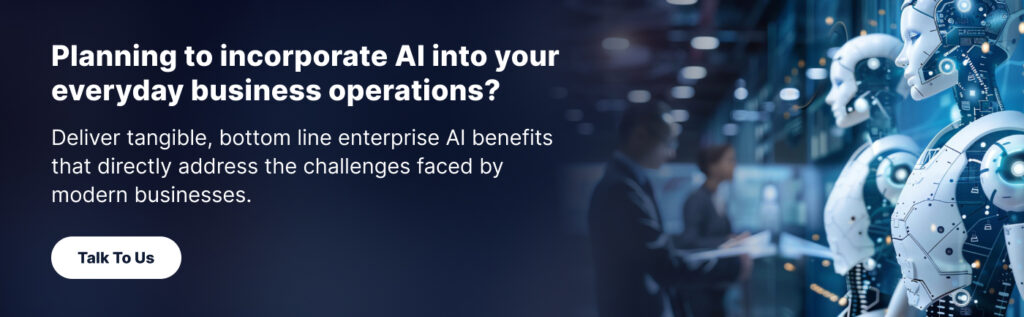