The future of R&D in Life Science hinges on using intelligent technologies to dramatically improve cycle times, success rates, and efficiency. A McKinsey study finds Generative AI (GenAI) could generate $60 billion to $110 billion annually in economic value for pharmaceutical and medtech companies with its ability to ability to predict off-target effects, optimize drug safety profiles, and incorporate digital tools for remote monitoring. In this blog, we delve into how GenAI is not just a tool but a transformative force driving breakthroughs across life sciences. Dive in to explore the trends shaping this revolutionary shift.
Generative AI in Life Sciences: What’s Trending?
Let us check out some prominent trends impacting the life sciences industry powered by GenAI.
Generative AI Permeates Every Discipline of Life Sciences
GenAI is revolutionizing the life sciences sector, becoming a key tool for innovation and efficiency. Deloitte reports that over 90% of leaders in biopharma and medtech recognize its transformative impact, with 66% of life sciences companies actively leveraging it. GenAI enhances operations by automating compliance processes, reducing errors, and ensuring regulatory adherence. Pharma supply chain management applies predictive analytics to anticipate demand and streamline inventory, improving logistics and distribution. This trend is set to grow, reshaping the industry in 2024 and beyond.
A Surge in Interdisciplinary Collaboration
GenAI encourages partnerships between life sciences companies and technology firms, leading to interdisciplinary collaborations that leverage expertise from both sectors. One of the standout healthcare trends for 2024 is the rise of interdisciplinary collaborations. Historically distant industries, like big pharma and digital health, are now uniting to improve healthcare delivery. Pharmaceutical companies are increasingly integrating digital solutions into their operations, marking a significant shift in approach. GenAI-integrated platforms enhance communication among interdisciplinary teams by providing shared virtual environments where researchers can collaborate in real-time.
For instance, Novo Nordisk partnered with Kakao Healthcare to offer advanced digital health services for managing chronic illnesses. Similarly, major players like Gilead, Merck, and Bayer co-founded the Digital Pharma Circle (DPC) to foster discussions on leveraging digital technologies to transform life sciences. This shift reflects a long-awaited step toward a more interconnected and innovative healthcare system.
Advanced AI and ML Technologies are Transforming Research
The integration of AI and machine learning in healthcare research is more than a trend—it’s a transformative revolution. Tasks that once took weeks or months, like data analysis, are now completed almost instantly with advanced AI and ML applications like GPT and LLMs. By processing millions or even billions of records simultaneously, GenAI is expediting drug discovery, improving medical trial recruitment, and enhancing disease detection. This leap in efficiency and capability is reshaping the landscape of life sciences and redefining the future of healthcare innovation. GatorTronGPT, a clinical LLM trained on extensive clinical data, has demonstrated improved performance in biomedical natural language processing (NLP). It can generate synthetic clinical text that aids in training other NLP models, thereby enhancing the overall analysis of clinical data without compromising patient privacy. Researchers leverage LLMs like ChatGPT to generate summaries of medical literature to upgrade their knowledge or accelerate documentation.
Data Becoming a Key Player in Research
Data plays a key role in medical research, and it’s becoming the driving component of AI-driven initiatives in Life Science research. As AI and ML models need to train on tons of data to become capable of delivering expected outputs access to high-quality data from various disciplines is essential. GenAI techniques can augment clinical research datasets by generating synthetic data, which helps overcome limitations related to data scarcity and privacy concerns. Synthetic datasets maintain statistical validity while protecting patient privacy, enabling broader access to valuable research data. Collaborative platforms that utilize GenAI enable scientists from various disciplines to share insights derived from integrated datasets, promoting a multidisciplinary approach to tackling complex health challenges.
Use Cases of Generative AI in Life Sciences
GenAI leverages deep learning neural networks inspired by the structure of the human brain to revolutionize life sciences. These networks consist of layers of interconnected nodes that process data, such as medical images, patient records, or genetic sequences. As data flows through the layers, each node refines it step by step. The output, produced in the final layer, can include medical visuals, drug formulations, or scientific publications. This adaptable technology generates content in various formats, offering immense value across the life sciences industry. Let us focus on the top use cases of GenAI in Life Sciences.
Drug Discovery
GenAI is transforming drug discovery by accelerating the identification and development of novel therapeutic compounds. Traditional drug discovery processes can be time-consuming and costly, often taking over a decade and billions of dollars to bring a new drug to market. Using algorithms trained on vast datasets of chemical structures and biological interactions, GenAI can propose new drug candidates with desired properties. GenAI models can analyze existing data to predict how new compounds will behave in biological systems, helping researchers identify potential safety concerns early in the development process. By simulating variations of known compounds, GenAI can suggest modifications that enhance efficacy or reduce side effects, thereby expanding the chemical space explored during drug discovery.
Synthetic Gene Design
In the field of synthetic biology, GenAI is playing a pivotal role in designing genes with specific functions. Generative algorithms can design synthetic genes that encode proteins with desired characteristics or functions, facilitating advancements in gene therapy and biotechnology. By predicting how changes in genetic sequences will affect protein production and function, GenAI helps optimize gene constructs for research or therapeutic applications. GenAI tools allow researchers to explore a broader range of genetic modifications rapidly, enhancing their ability to develop innovative solutions for genetic disorders.
Single-cell RNA Sequencing
GenAI is revolutionizing single-cell RNA sequencing (scRNA-seq) by enhancing data analysis methods used to understand cellular diversity within tissues. Generative models can integrate scRNA-seq data with other omics data (e.g., proteomics) to provide a comprehensive view of cellular functions and interactions. By analyzing complex datasets generated from scRNA-seq experiments, GenAI helps identify distinct cell types or states within heterogeneous populations, advancing our understanding of diseases like cancer. Generative models can propose novel hypotheses regarding cellular behavior based on observed patterns in scRNA-seq data, guiding future experimental designs.
Data Augmentation for Model Training
GenAI plays a crucial role in augmenting datasets used to train machine learning models in life sciences. Generative models can produce synthetic datasets that mimic real-world data distributions while preserving essential characteristics. This is particularly useful when real-world data is scarce or difficult to obtain due to privacy concerns. By providing diverse training examples through synthetic data generation, GenAI helps improve the robustness and generalizability of machine learning models used in diagnostics or predictive analytics. In scenarios where certain conditions are underrepresented in training datasets (e.g., rare diseases), GenAI can create additional examples to balance classes effectively, leading to improved model performance.
Conclusion
GenAI is making significant strides across various wings of Life Sciences by enhancing drug discovery processes, facilitating synthetic gene design, and augmenting datasets for model training. By leveraging high-quality integrated datasets and GenAI techniques, researchers can unlock new insights that drive innovation and improve patient outcomes. As these technologies continue to evolve and integrate into healthcare practices, they hold tremendous potential to drive innovation and improve outcomes across the life sciences sector.
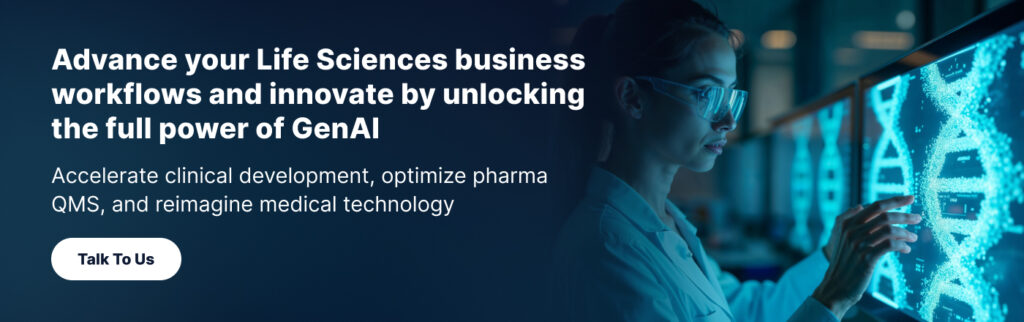