Since the launch of ChatGPT the generative AI has started a tech revolution with astonishing momentum. Enterprises are in a quest to optimize almost every workflow leveraging generative AI applications. This leads us to ponder what’s the next frontier of generative AI evolution.
Multimodal AI, an ML model, seems to be a promising evolution of Generative AI capable of combining inputs of various modalities like text, images, videos, or audio clips and creating an output that may also be multimodal.
Why Multimodal AI Is the Future?
The pinnacle of AI evolution is achieving Artificial General Intelligence (AGI) a hypothetical AI system that can understand, learn, and apply knowledge across a wide range of tasks, much like a human. The first modern generative AI models, like ChatGPT, are unimodal. Most of these models process text prompts to generate text-based responses. To achieve AGI a model should be able to function like a human brain relying on the five senses for collecting all kinds of information from the surrounding environment.
Reading text is just one of the various ways to learn new things. Multimodal learning emphasizes augmenting the learning capacity of training models via text, as well as other sensory data types such as images, videos, or audio recordings. This enables models to discover new patterns and inferences by building correlations between text-based metadata and their associated images, videos, or audio.
This ability of cross-modal understanding of multimodal AI unlocks immense possibilities like generating an image from a written description, composing an underlying musical track from a sequence of images, or providing automatic dubbing of videos into different languages. Ensuring coherence between different modalities requires a bit of complicated architecture and in the next section, we are going to explore that.
The Basics of Multimodal AI Architecture
Multimodal generative AIs are built on a type of neural architecture called a Transformer. For sorting text, grasping context, and generating new text BERT (Bidirectional Encoder Representations from Transformers) and GPT (Generative Pre-trained Transformer) lead the pack. For recognizing, classifying, and generating new images we use CNNs (Convolutional Neural Networks) and Vision Transformers. Speech recognition and audio synthesis can be performed by DeepSpeech and WaveNet, respectively.
For better predictions, multimodal AI relies on data fusion to combine the complementary information that different modalities of data provide. Data fusion techniques can be broadly categorized under three types depending on the processing stage.
Early Fusion
Early fusion, or feature-level fusion, involves combining data from multiple modalities at the input stage before any significant processing occurs. This means that features from different sources are merged into a single representation successively fed into the model.
Mid Fusion
Mid fusion, or intermediate fusion, processes each modality into a latent representation to make them fit for fusion before feeding the model for final processing.
Late Fusion
Late fusion, also known as decision-level fusion, processes each modality independently using separate models or feature extractors. The outputs or decisions from these models are combined at a later stage to make a final prediction.
The Benefits of Multimodal AI
Multimodal AI offers substantial and diverse benefits across various applications and industries. Let’s gain a comprehensive understanding of these advantages provided.
Enhanced Contextual Understanding and Interpretation
Understandability of both language and visuals enables multimodal AI to have a broader context of things. Compared to interpretation gained from just text-based data analyzing text, images, videos, and sound gives a comprehensive interpretation and far accurate interpretation of contexts.
Richer and Nuanced Content Creation
Multimodal AI responds with complex and rich content outputs blending more than one modality. For example, they can create a complete video with all the sounds and subtitles based on text prompts. GPT-4V (GPT-4 Vision) a multimodal model offers hyper-realistic interactions by engaging in a conversation when a user uploads an image. Another interesting application of multimodality is in marketing where the model can create the complete content for a social media post along with images, text opener, and hashtags.
Efficient Data Fusion
The ability to merge information from different sources enables multimodal AI to provide a more comprehensive view of complex situations. For instance, in marketing, this technology can help businesses evaluate customer feedback in different formats, such as text reviews, video reviews, and social media posts on diverse platforms. The ability to mix facial and speech recognition enables them to comprehend complex queries and commands, leading to enhanced user satisfaction and effective usage of technology.
Enhanced Compliance and Threat Detection
Multimodal AI interprets a context from different modalities of content. By merging information from varying sources across multiple modalities they can identify and handle inappropriate or harmful content across platforms that use diverse media forms. This property is useful in areas like surveillance and threat detection and maintaining regulatory compliance.
Applications of Multimodal AI
The adoption of multimodal AI is spreading across industries. Check out this potential use case.
Healthcare
Clinical Diagnosis: AI systems draw information from different modalities of medical documents. They study CT Scans, X-rays, and at the same time check out radiology and pathology reports, digital data from monitoring devices, and compare them with previous prescriptions stored as EHR to trace a comprehensive pattern and increase the accuracy of diagnosis.
Patient Interaction: Multimodal AI adds a human touch while interacting with patients. They can respond to voice or text prompts from patients and retrieve an EHR or suggest something to resolve their concerns. They can also help patients understand the nature and cause of the ailment through illustrative diagrams, and AR models or help them complete the paperwork for admission or insurance claims.
Manufacturing
Multimodal AI learns from sensory data, camera visuals, and machinery sounds for predictive maintenance of equipment. Autonomous vehicles are common in factory floors and warehouses for transporting manufactured goods and raw materials. Multimodal AI processes data from sensors, cameras, radar, and lidar to enhance real-time vehicle navigation, interpret the presence of hazards or factory workers on the path, and ensure seamless driving through complex scenarios.
Finance
In the banking and finance sector, multimodal AI analyzes diverse data types, such as transaction logs, user activity patterns, for risk management and fraud detection. For example, an AI-based system can check credit score and analyze the social media activities of a loan applicant to assess his financial stability. JP Morgan’s DocLLM combines text data, metadata, and contextual information from financial documents for automatic document processing and risk evaluation.
ECommerce
A major motive behind multimodal AI is to provide personalization and enhance customer experience. In eCommerce AI chatbots and virtual assistants build context from webpage interaction, social media reviews, and customer queries to generate personalized product recommendations or respond empathetically to customer complaints. Multimodal AI also helps to improve customer service through content generation for customer care agents browsing the enterprise knowledge base. An interesting application is Amazon’s packaging where a multimodal AI blends information about product size, shipping instructions, and environmental regulatory guidelines to improve packaging precision and aim for sustainability.
Energy & Utilites
Multimodal AI combines data from operational sensors, geological surveys, and environmental reports for precision in mining or energy demand prediction. ExxonMobil leverages a multimodal AI to predict equipment needs, optimize drilling operations, and respond swiftly to environmental changes.
Conclusion
Multimodal AI is a promising technology with its ability to integrate diverse data types into a single AI model. As generative AI and language models evolve, more sophisticated data integration from different modalities will become a possibility. Recognizing social cues in a conversation or adapting to environmental changes in real time will become the primary qualities of a multimodal AI. By understanding and utilizing this powerful tool, professionals and creatives alike can unlock unprecedented levels of innovation and efficiency.
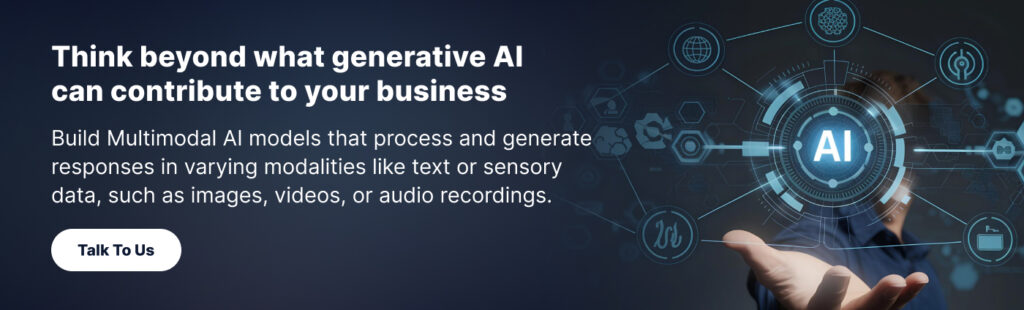