Healthcare is inclined to adopt the age-old adage of “prevention is better than cure”, and predictive analytics is making that possible. By leveraging historical data, machine learning, and AI, healthcare organizations can forecast trends, personalize treatment, and revolutionize patient care in ways we’ve only just begun to explore.
Through data-driven predictions, healthcare shifts from reactive to proactive, enhancing patient care and overall quality. By actively integrating advanced analytics and modeling, healthcare providers can forecast patient needs, spot emerging health trends, and create personalized interventions. It also strengthens risk management, allowing organizations to prevent adverse outcomes and improve care. In this article, we will investigate the transformations predictive analytics have to offer for the healthcare industry.
The Transformations Driven by Predictive Analytics in Healthcare
Delivering Personalized Care for Patients
Predictive analytics in digital health transforms patient care by enabling personalized, proactive interventions. Predictive models actively analyze patient data, diagnostic results, and treatment histories to predict health risks and identify individuals who may be at risk. This empowers healthcare providers to implement targeted interventions, preventing negative outcomes. By anticipating disease progression and fine-tuning treatment plans, clinicians can provide care tailored to each patient’s specific needs, preferences, and conditions, ultimately improving treatment outcomes and patient satisfaction.
Predictive analytics shifts healthcare toward a patient-centric approach by using data-driven insights to make decisions. Providers actively leverage predictive models to identify patients who will benefit most from a specific treatment protocol. Personalized medical intervention plans check disease progression and lower hospital readmissions, improving patient experiences. By keeping patients at the core of care and using predictive data to drive decision-making, healthcare organizations improve outcomes while promoting patient engagement and empowerment.
Transforming Healthcare Operations
Predictive analytics actively enhances operational efficiency in healthcare by forecasting patient volumes, resource needs, and identifying bottlenecks. This allows healthcare systems to streamline workflows, optimize resource allocation, and boost productivity. With data-driven insights, organizations can cut costs while ensuring that services are delivered more effectively and efficiently, benefiting both patients and healthcare providers alike.
Predictive analytics offer real-time insights that guide proactive decisions in healthcare. By leveraging predictive models, organizations can forecast patient demand, adjust staffing, and allocate resources more effectively. It also uncovers inefficiencies, driving continuous improvements and cost-saving measures. Using data to inform operational strategies, healthcare systems can enhance efficiency, reduce waste, and improve the overall quality of care.
Advancing Preventive Healthcare Practices
Predictive analytics is the catalyst for preventive healthcare practices. Using predictive models and population health analytics, healthcare organizations can develop targeted preventive measures, early interventions, and wellness programs to reduce risks and promote healthier outcomes. This proactive strategy encourages individuals to manage their health more effectively, fostering a culture of prevention and community well-being.
Predictive analytics drives preventive healthcare by identifying individuals at risk of chronic conditions or adverse health events. Using predictive models and risk stratification, healthcare providers can target high-risk populations and implement interventions to improve outcomes. Predictive analytics also supports population health management by allocating resources based on predicted needs, maximizing impact, and enhancing community health. By adopting this proactive approach, healthcare organizations can lower costs, improve health outcomes, and promote a culture focused on wellness and prevention.
Challenges Associated with Adopting Predictive Analytics in Healthcare
Arousing the Doctor’s Interest
Healthcare providers are pressured continually to enhance their digital skills. Predictive analytics, where they need to access dashboards and manage patient data, seems to be a new learning curve. Balancing data collection and patient care during appointments poses challenges. To address this, medical facilities can involve staff in the development of predictive analytics tools, ensuring their feedback shapes solutions that improve workflows and ease the integration of data collection into patient care.
Moral and Ethical Challenges
People often take more risks when they feel backed by safety measures, and this can happen in healthcare when doctors overly rely on predictive analytics, assuming these tools bear full responsibility for outcomes. To counter this, it’s essential to reinforce that analytical tools provide guidance, not absolute answers. Clinicians should critically assess the recommendations and, when appropriate, engage patients in the decision-making process to ensure thoughtful, well-rounded care.
Algorithmic Bias
Algorithmic bias can impact a model’s effectiveness on certain datasets, and the lack of clear regulations on algorithm development makes this issue more challenging. Currently, ensuring fairness in predictive tools is the vendor’s responsibility. To minimize bias, vendors can implement feedback loops to refine their tools, while healthcare organizations should conduct regular audits to confirm that algorithms remain relevant and unbiased over time.
Model Explainability
Many advanced healthcare predictive analytics models operate as black boxes, meaning they don’t reveal how they generate their outputs. While this is acceptable for administrative tasks, such as predicting rejected insurance claims, doctors are unlikely to trust recommendations impacting patient care without understanding the reasoning behind them. A viable solution for clinics is to explore explainable AI, which clarifies the rationale behind predictions in cases that directly affect patient health.
Use Cases of Predictive Analytics in Healthcare
Predicting Disease Outbreak
BlueDot, a Canadian company building predictive analytics and AI solutions, issued a warning about the rise of unfamiliar pneumonia cases in Wuhan on December 30, 2019. Only nine days later, the World Health Organization released an official statement declaring the emergence of the novel coronavirus.
Forecasting Appointment No-Shows
A team of researchers cooperated with Doctor Luis Calvo Mackenna Hospital, a pediatric facility in Chile, which reported a high no-show rate of 29%. The researchers used healthcare predictive analytics and ML to process patient data, such as demographics and social conditions, to spot patients prone to no-shows and call them with a reminder. During the eight-week experiment, the researchers managed to reduce no-shows by 10.3%.
Identification of At-risk Patients
UnityPoint Health built a predictive model for assessing the readmission risk score of every patient. The tool allows senior physicians to predict and prevent a patient’s readmission by starting early treatment of symptoms. The tool was able to reduce readmission rate by 40% within 18 months of implementation.
Conclusion
Predictive analytics is a key driver of digital transformation in healthcare. It offers a significant contribution in various areas of the healthcare industry from optimizing patient care to accelerating clinical trial in drug discovery. The foundation of powerful predictive analytics lies in data transformation, good quality data, and AI models rigorously trained on transformed data. The complexity of building a reliable predictive analytics model can be overwhelming for healthcare businesses and they might consider looking for an experienced AI and datascience partner to analyze their objectives and lay out a technical roadmap while the core team can focus on improving the condition of healthcare services.
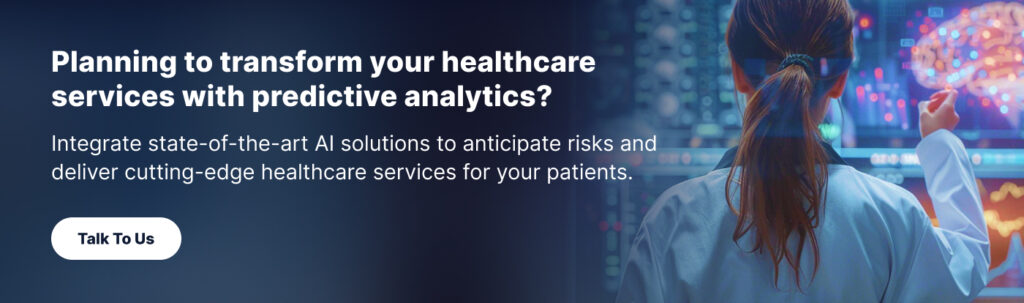